Cloud has been in existence since 2006, when Amazon Web Services (AWS) first announced its cloud services for enterprise customers. Two years later, Google launched App Engine, followed by Alibaba and Microsoft’s Azure services. The most recent addition to the public cloud service providers’ list is OCI (Oracle Cloud Infrastructure).
As per the Gartner 2021 Magic Quadrant, AWS is the market leader, followed by Microsoft Azure and Google Cloud Platform in the second and third positions, respectively. As cloud technology evolves, so do the customer requirements. Today, cloud adoption is one of the top priorities among C-suite executives. The Covid-19 pandemic further accelerated the need for cloud adoption as digitalization is no longer optional for organizations but a mandate. As the pandemic nears its end, there is a surge in demand for cloud services as most enterprises are increasingly leveraging it. As a result, enterprises don’t spend enough time on the “right” workload assessment. There is a possibility that enterprises might get impacted due to this sudden move to the cloud and may have to eventually exit or switch to another Hyperscaler at a later stage.
As per Gartner’s report, 81% of the respondents said they currently work with two or more public cloud providers. It means multi-cloud is the future of cloud computing.
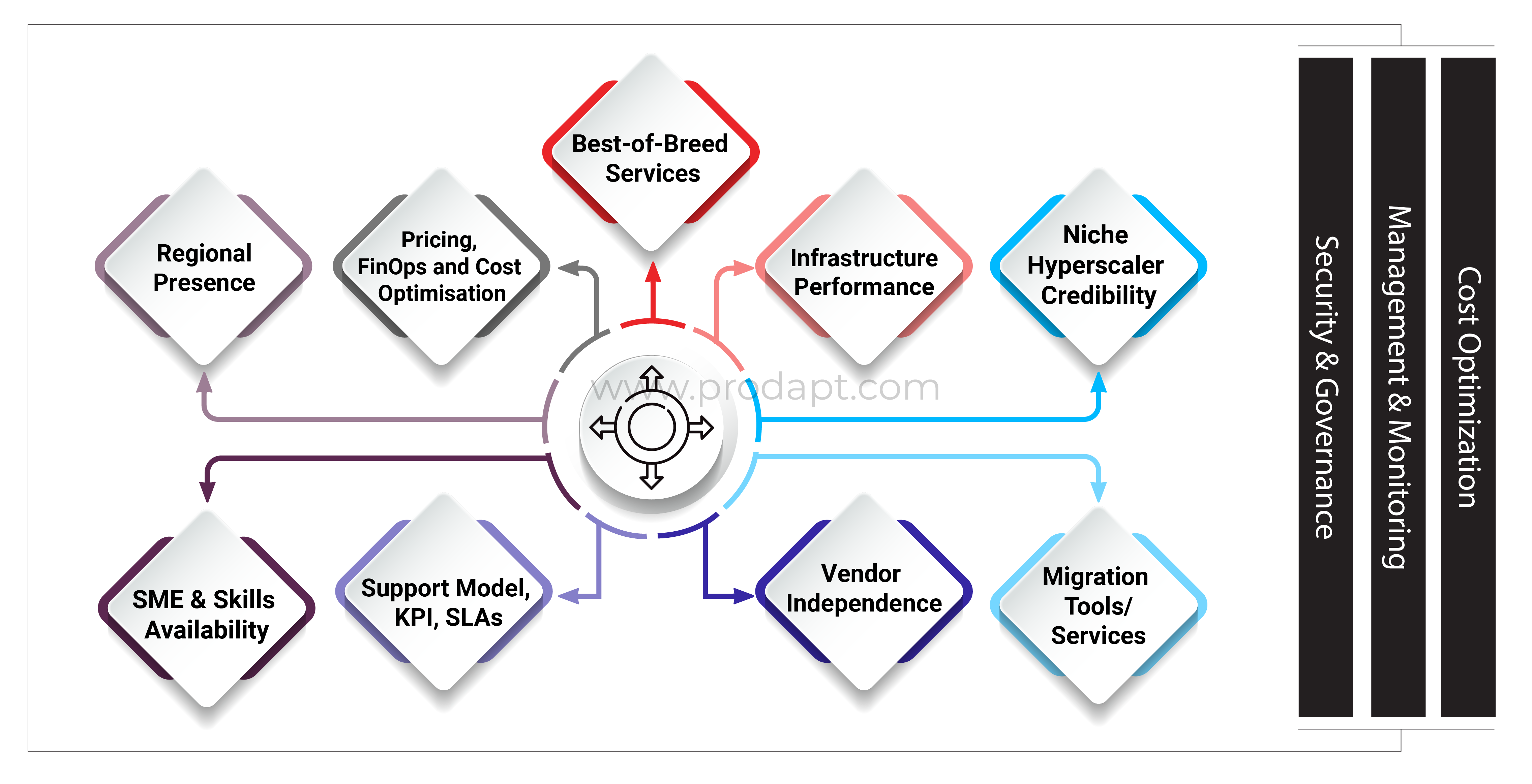
- Regional Presence – This is one of the most common requirements when selecting the Hyperscaler. Most well-known Hyperscalers have extended their global reach to tap into new markets, meet existing customer demands and adhere to regulatory/compliance requirements. Regional presence has a strong impact as enterprises would prefer being closer to their customers, abide by the compliance requirements defined by their country and offer high performant services with low latency. When planning to onboard another Hyperscaler, enterprises must ensure that it fulfils all the regulatory and compliance requirements and has a presence in the local region. Additionally, enterprises must perform a small proof of concept if switching due to latency-related reasons. Besides, they must also evaluate the connectivity options available through Hyperscaler or their Channel Partners.
- Best-of-Breed Services – All major Hyperscalers offer a huge portfolio of services across infrastructure, platform, data services, and AI/ML. Yet, some cloud service providers enjoy market leadership for specific services. Enterprises can go for any Hyperscaler for general infrastructure. However, large enterprises, majorly depending upon Microsoft technologies and tools, prefer Azure, as they get to leverage the Microsoft Licensing Model and ease of integration. Lastly, GCP becomes the vendor of choice among enterprises regarding AI/ML/Data services. When evaluating another Hyperscaler, enterprises must validate new and different services that are available with the new Hyperscaler. Evaluate these services for proper functionality, limitations, resource limit, and availability in the chosen region. For a Hyperscaler, all services may not be available in all the regions. Review the Hyperscaler’s roadmap and ensure that the required services will be available before the switch-over.
- Vendor Independence – Vendor/cloud provider lock-in can be extremely detrimental, keeping you captive for non-competitive pricing. It can also impact your agility, productivity, and growth if a cloud provider is failing to live up to the committed SLA terms and you are prevented from switching to another provider. Opting for a multi-cloud strategy early in the cloud journey would help enterprises avoid getting locked into such vendor dependence. There are different models today, like using generic services from one Hyperscaler and specialized services from another and using one Hyperscaler for production workload and another for disaster recovery. Enterprises should ensure that the applications can work across different clouds before finalizing the strategy, especially for stateful applications.
- Infrastructure Performance – Every Hyperscaler has built its environment using different virtualization technology called a hypervisor. While AWS uses Xen hypervisor for the old generation and Nitro Hypervisor for the newer generation, Oracle Cloud Infrastructure uses Xen technology, and Google Cloud Platform uses KVM. In addition, their services are hosted on the latest and greatest hardware stack. There is a possibility that some workloads may perform slightly better in one environment than another due to abstraction overhead or the underlying new hardware. Also, some Hyperscalers offer different hardware in different regions, so enterprises need to assess this based on the application they plan to deploy in a region. As a recommendation, enterprises can perform a Proof of Concept (PoC) by running the same application across different Hyperscalers. This may require running the same workload in the new setup for a specific duration and closely monitoring it. Try simulating the same use case, setting up alerts, gradually increasing the use-case traffic, and monitoring the application behavior. Based on the PoC results, host your applications across multi-clouds.
- Niche Hyperscaler Credibility – There are options beyond the major Hyperscalers that might fit into enterprise niche needs. It is critical to validate these niche vendor’s credibility during the evaluation phase. Enterprises can make use of third-party services to ensure vendor credibility. Industry analysts like Gartner, IDC, Forrester, etc., regularly publish vendor-oriented reports. Look out for their evaluation of the Hyperscaler in Magic Quadrant, Forrester Wave, etc. The Hyperscaler must have a long-term strategy, plan, and roadmap.
- Migration Tools/Services – For an enterprise planning to onboard another Hyperscaler, it becomes equally important to select the right tool to migrate the workloads from on-premises to cloud or from one Hyperscaler to another. For this reason, evaluate if the new Hyperscaler provides any tools or services for workload, database, and data migration to their environment.
For example, every Hyperscaler has a set of tools for workload migration, database migration, data migration, data transformation, etc. AWS provides Application Migration Services for workload migration, AWS Database Migration Service for database migration, AWS DataSync for data migration from on-premise to AWS. Similarly, Google Cloud Platform has tools to make the data and workload migration very seamless – Migrate for Compute Engine for workload migration from On-Premise to GCP, AWS/Azure to GCP (Hyperscaler to another Hyperscaler), Migrate for Anthos for workload transformation from GCE to GKE, AWS EC2/Azure VM to GKE (one Hyperscaler to another Hyperscaler) or Storage Transfer Service for Cloud, etc. Likewise, Azure has Azure Migrate for workload migration, Azure Database Migration Service for databases, etc.
- Pricing, FinOps, and Cost Optimization – Service consumption charges are always a top priority for a CFO. Enterprises are constantly exploring different options to reduce their operating expenses. They expect Hyperscalers to recommend options to reduce cost, display granular usage and report service-wise breakdown. Tools/platforms like CloudCheckr, CoreStack (FinOps), Flexera CMP, etc., offer recommendations and insights for cost optimization. These products/tools use an advanced ML-based approach to the past (historical) data to recommend the next course of action. Cost optimization plays a vital role in deciding the multi-cloud strategy.
- Support Model, KPI, SLAs – Few enterprises may also want to add another Hyperscaler since the available Hyperscaler cannot meet the required SLA or they don’t offer well-defined KPIs. These are a few key measurable parameters for an enterprise to discuss with their Hyperscaler before deciding. It helps in evaluating the cloud partners, measure the project progress and its impact on their business. Evaluate the benefits of each support model available through the Hyperscaler. Go for the one that best suits the enterprise’s requirements. Check different SLAs, KPIs, monthly/quarterly reports, etc.
- SME & Skills Availability – For going multi-cloud, an enterprise will require guidance at every stage, like identifying the right workloads, right Hyperscaler(s), right monitoring and management tools, right skills, etc. For these reasons, an enterprise must have or engage an expert or a system integrator (SI) who can advise, help the team and guide them through the multi-cloud journey. In addition, define a path for the internal teams to learn new skills and get certified
As the public cloud offerings and services expand, enterprises have multiple options available at their disposal. They can decide and pick up the most suitable Hyperscaler for their workloads. Workload mobility across clouds will be a general pattern based on service cost, application latency, and/or need for additional resources. Though it may not be ideal for critical production-grade workloads/applications with regulatory and compliance requirements, it is most suitable for other workloads like product testing, scalability testing, code development, etc., which caters to around 30%-40% of the workloads. Such workloads can make use of this capability to achieve cost optimization.
Earlier, due to a limited number of cloud service providers, enterprises had to worry about service outages, vendor lock-in, delays in problem resolution, vendor insolvency, etc. But with the blooming Hyperscaler eco-system, enterprises are flooded with choices. This leads to challenges in effectively managing, monitoring, securing, and optimizing costs in a multi-cloud environment. However, enterprises can use multi-cloud management solutions from vendors like IBM (Cloud Pak), Micro Focus (Hybrid Cloud Management X), Flexera (Cloud Management Platform), Scalr, ServiceNow (ITOM Cloud Management), etc. to ensure seamless operations.
A multi-cloud strategy also demands well-defined governance. Otherwise, it may increase the operating costs due to ignorant individuals or poor control mechanisms. An inefficient governance (control mechanism) may lead to underutilized and zombie resources, consuming money in the cloud. It is recommended to set up a central body responsible for managing the cloud resources and ensuring proper governance. Creating a self-service portal with proper workflow is a good approach to managing the cost and handling mismanagement.
Today, we are already consuming “serverless” services from cloud service providers, but, in the future, we may have a new business model where the enterprises pay for the services and forget worrying about where exactly it’s hosted. In the current product market, acquisition is a common strategy adopted by companies to expand their customer base, add unique services to their portfolio, and/or enhance their capabilities. Tomorrow, the trend may continue among the Hyperscalers too. Who knows what’s next in the technology roadmap?