According to Nielsen, “The right solutions could save up to $1.6 billion of revenue lost to customer churn in a year.”
Introduction
The Connectedness industry has encountered various challenges throughout its existence, with one major challenge being customer churn. Customer churn refers to the percentage of customers who terminate their subscriptions or switch to a different telecom provider within a specified time limit.
The Connectedness industry experiences the highest customer churn rate compared to other sectors. According to a study by the Aberdeen Group, the average telecom company loses $100 per month for every customer that churns. This means that a company with a 10% churn rate could be losing $120 million per year.
According to Forrester, a bad experience is enough to prompt customers to consider switching providers. This makes continuous investment in customer churn prediction necessary, given that customer retention is more cost-effective than customer acquisition. High customer churn has detrimental effects on the business, including revenue loss, missed cross-selling and upselling opportunities, and difficulties in forecasting and planning for future growth.
The emergence of data collection techniques and the need to generate deep insights have led to the expansion of analytics applications across several domains. However, Communications Service Providers generate vast amounts of data every day, making it a significant challenge to draw meaning out of such complex, multi-faceted data.
Customer churn analysis is resource-intensive and requires extensive computational power
As the volume and type of data captured in the Connectedness industry increase exponentially, the number of metrics and evaluations that require processing also increases. Customer churn prediction and analysis are usually carried out using ML modeling. Numerous attributes are used for research, such as the billing data, Call Detail Records (CDRs), and Contract/Subscription data. Hence, customer churn prediction requires significant computational power.
Furthermore, customer churn is a complex process that involves multiple interdependent parameters. For instance, network quality, which directly affects customer satisfaction, can be impacted by several other factors, such as network congestion, signal strength, and coverage area. A recent Gartner report predicts that enterprise-generated data processed in data centers, or the cloud, will increase to 75% from the current 10% by 2025. In other words, more than 180 zettabytes of data will be generated globally from over 41 billion connected devices. As more parameters are added to make precise predictions, the current predictive methods will become ineffective in processing and analyzing the multi-faceted and intricate data, which require extensive time, energy, and resources.
Major challenges with the Classical Machine Learning1approach of churn prediction
- Examining large and diverse datasets to provide personalized solutions is a challenging task that often results in resource wastage
- Allocation of resources is challenging due to the shift from a centralized to a
hyper-distributed subscriber environment
- Analyzing and deriving insights from multidimensional data is cumbersome, leading to difficulties in identifying and extracting complex churn patterns
Service providers must embrace Quantum Machine Learning to overcome the shortcomings of Classical ML, such as slower processing times, inability to process large amounts of data in parallel, and low accuracy.
Quantum Machine Learning (QML): A strategic imperative to predict customer churn and maintain the competitive edge
Quantum Machine Learning (QML) offers a new approach to analyzing large datasets and extracting valuable insights for faster estimation of customer churn. It can efficiently model high-dimensional feature space using quantum parallelism. Quantum parallelism is a feature of quantum computers that allows them to perform multiple calculations simultaneously, exploiting the superposition of quantum states to explore multiple solutions at once. By leveraging the power of quantum computing, it can perform brisk calculations, enabling businesses to analyze customer data efficiently and effectively.
Using QML, service providers can develop faster predictive models to identify customers likely to churn. These models can process factors such as customer demographics, purchase history, and browsing behavior, thereby increasing the efficiency of finding at-risk customers.
Here are three scenarios where QML can excel:
- Complex pattern recognition: Churn prediction requires identifying intricate patterns and dependencies in the data, which is difficult for Classical ML. Quantum ML can be leveraged to handle complex computations and analyze high-dimensional data, including call frequency, data usage, location, and customer demographics, to uncover hidden correlations contributing to churn.
- Real-time churn Prediction: Preventing churn requires timely action, and Classical ML, due to its slower processing time, proves ineffective in this regard. Quantum ML enables real-time churn prediction by providing faster computations and optimizations. It can process data quickly, allowing service providers to identify potential at-risk customers and take proactive measures to retain customers promptly.
- Handling Big Data: Churn prediction often deals with large datasets that can be computationally intensive to process using Classical ML methods. QML can provide computational advantages for analyzing big data by leveraging the inherent parallelism and quantum algorithms designed for data-intensive tasks. Telecom marketing leaders can use QML to fine-tune models for predicting churn and optimizing parameters like learning rates and regularization factors for improved accuracy without extensive trial-and-error experimentation.
Furthermore, QML could help service providers explore new data analysis and predictive modeling possibilities, potentially leading to accurate insights from the available data. It will allow data teams to explore intricate data relationships, enhance security measures, and handle significant data challenges.
The following table talks about a sample customer churn prediction model, highlighting the advantages of Quantum ML over Classical ML in computing highly complex and interdependent data.
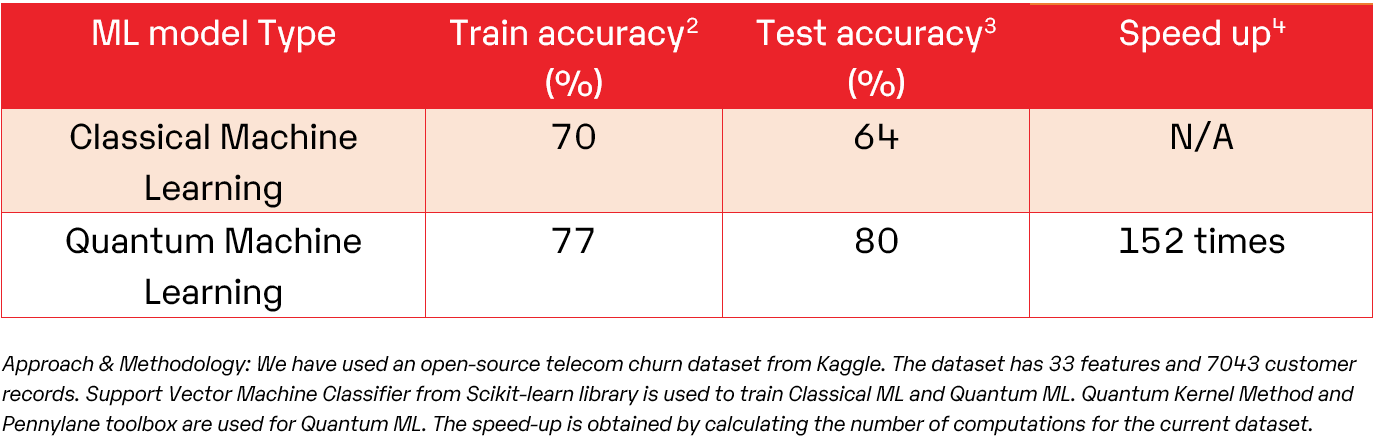
Table 1: Comparison of Classical ML and Quantum ML implementation of customer churn prediction
A notable enhancement in the overall speed, by 152 times, indicates a significant advancement in both computational efficiency and analysis of extensive datasets. This progress highlights the rapid evolution of QML capabilities and underscores the potential to tackle complex problems and derive insights faster.
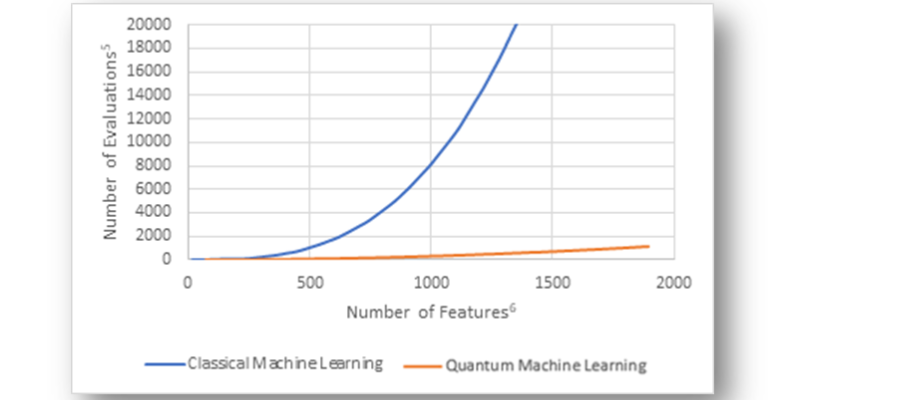
Graph 1: Scalability7 potential of Classical ML & Quantum ML
As the number of parameters within a dataset expands, implementing QML significantly reduces the overall number of evaluations required for data processing. In the graph, as the number of features/parameters increases, the number of assessments increases exponentially for Classical ML, signifying that more computational power and resources are required to solve a highly complex problem. In contrast, the graph is relatively flat for Quantum ML, indicating that it will be highly efficient in solving complex problems.
Given the widespread anticipation of a big data surge across industries, the escalation in the number of prediction parameters is inevitable. This makes QML’s efficiency imperative for service providers to enable them to save time and drive decision-making capabilities.
Conclusion
Quantum ML is close to a breakthrough in its journey. It can completely reform the machine learning process and models, drastically reducing processing time and significantly improving performance. Leveraging QML can enable service providers compute complex use cases like customer churn instantly and accurately, providing considerable benefits to the service providers.