Insights / Digital Customer Experience
Making of an intelligent Virtual Agent to transform Customer Experience
Leverage a Machine Learning-based approach for optimizing Virtual Agent Training to improve its precision, recall and accuracy
Virtual agents are an integral part of businesses with an online presence as they provide round-the-clock assistance to customers. They augment teams to enable a rich experience for both customers and live agents.
The success of any Virtual Agent (VA) depends on its Natural Language Understanding (NLU) training, which should not be just a one-time activity before the configuration, but a continuous process. The challenge is to provide the right set of representative examples from historical data for the training. Identifying a few hundred sample examples from millions of historical data is a herculean task. Moreover, this task is often done manually. Thus, the task of finding the most suitable examples becomes questionable as well as extremely time-consuming.
Service Providers must develop a Machine Learning (ML)-based tool to identify the most appropriate and small data set of representative examples for training. The examples cover maximum scope for the respective intent, making NLU training highly efficient with improved precision, recall and accuracy. The ultimate benefit of this is improved customer experience, containment, and reduced abandonment. Since this is a tool-based approach, it also saves a lot of time in comparison to the manual process of identifying the training examples. Improved training efficiency in the first go also saves time and effort during subsequent re-training.
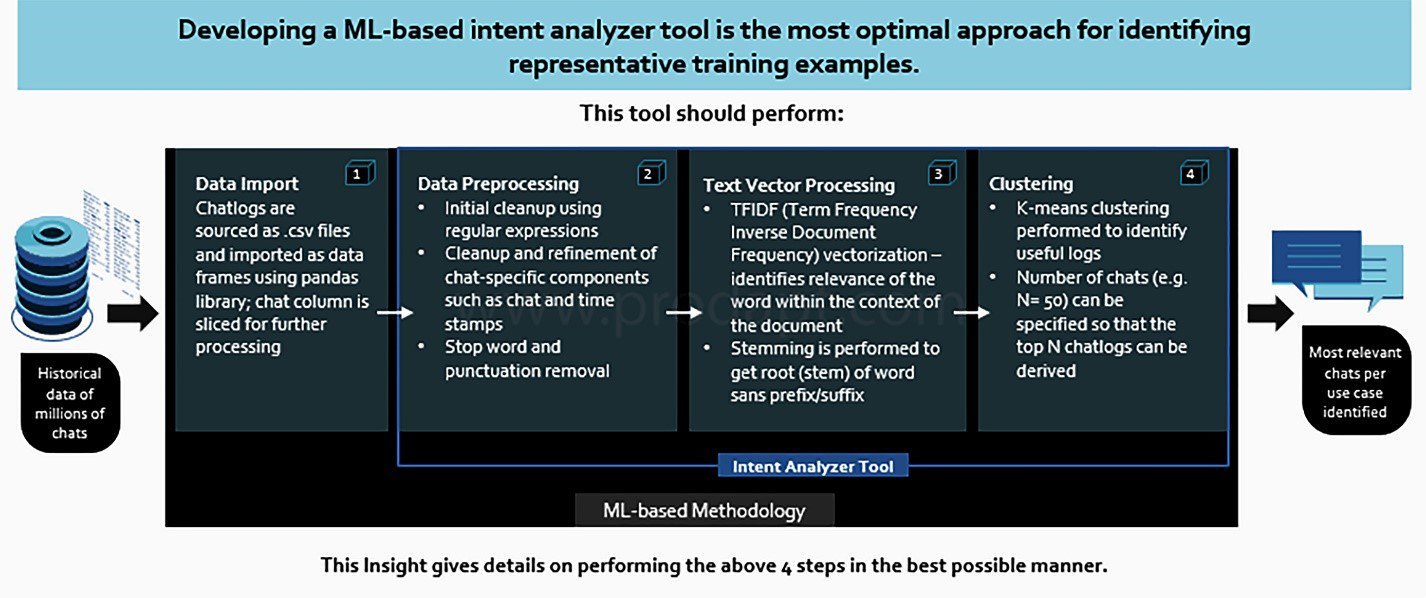
Figure 1: ML-based intent analyzer tool
VAs often fail to satisfy the customers due to their inability to identify the right intent. And this is the effect of wrong or inadequate training of VA’s natural language understanding (NLU) engine.